A Directors Guide To Building An Artificial Intelligence (AI) Roadmap
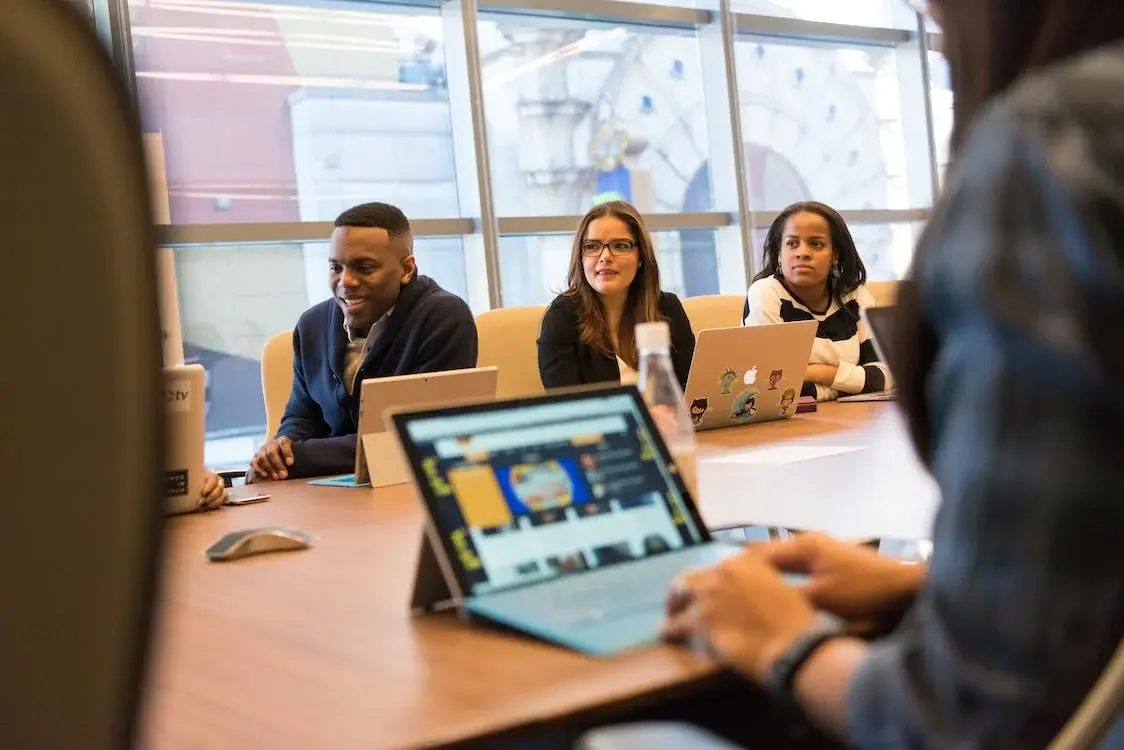
Artificial intelligence (AI) has rapidly shifted from a futuristic concept to a central pillar of business strategy. For CIOs and directors, the challenge is no longer whether to embrace AI, but how to do so in a way that delivers tangible, scalable business value. Building a robust AI roadmap is essential for organizations aiming to unlock the full potential of AI while aligning technology investments with strategic objectives and operational realities.
The Strategic Imperative for an AI Roadmap
AI’s transformative potential is widely recognized, but realizing its promise requires more than deploying new technologies. CIOs are tasked with translating the broad concept of AI into a concrete sequence of actions that drive business outcomes. A well-defined AI roadmap serves as a guiding framework, helping organizations align AI initiatives with business goals, manage resources effectively, and prioritize activities that matter most.
Unlike traditional IT projects, AI adoption is not a one-size-fits-all endeavor. Each organization must craft a roadmap tailored to its unique ambitions, maturity level, and operational context. The roadmap should be dynamic, evolving as the organization’s capabilities, needs, and the AI landscape itself change.
Seven Workstreams for Effective AI Implementation
A comprehensive AI roadmap organizes critical activities into seven interconnected workstreams. These workstreams provide structure and clarity, ensuring that all facets of AI adoption are addressed—from strategy to data, from governance to culture.
A Robust Strategy: The foundation of any AI initiative is a clear, succinct articulation of the organization’s AI ambitions. This involves defining the strategic impact AI is expected to deliver, in alignment with broader business goals. The initial strategy informs adoption goals and helps prioritize use cases. As the organization matures, the strategy should be refined and its success measured, ensuring ongoing alignment with evolving business needs.
An AI Value Assessment: Business value from AI is realized through a portfolio of initiatives. Early efforts typically focus on identifying and prioritizing use cases, running pilots, and demonstrating measurable value. Over time, organizations should move from isolated projects to a portfolio approach, continuously evolving AI products and services to meet changing customer and market demands. Tracking and communicating the value delivered by AI is crucial for sustaining executive support and investment.
An Organization Plan: Scaling AI requires more than technical expertise; it demands organizational readiness. Initially, this may involve forming a community of practice or dedicated teams focused on high-priority activities. As AI adoption grows, organizations should develop a target operating model that supports broader deployment, including clear roles, responsibilities, and processes for managing both internal and external partnerships.
People Considerations: Technology alone cannot drive AI success. Building a culture that embraces AI, encourages experimentation, and supports ongoing learning is vital. This includes upskilling employees, fostering cross-functional collaboration, and addressing concerns about AI’s impact on jobs and workflows. A strong AI culture accelerates adoption and helps manage the organizational change that AI inevitably brings.
AGovernance Framework: Responsible AI deployment hinges on robust governance. This involves establishing clear policies, frameworks, and oversight mechanisms to ensure ethical, secure, and compliant use of AI. Governance must evolve alongside AI capabilities, addressing issues such as data privacy, algorithmic bias, and transparency. A well-governed AI environment builds trust with stakeholders and mitigates risk.
Solid Technical Foundations: The technical backbone of AI initiatives, engineering encompasses architecture principles, design methodologies, and the selection of appropriate frameworks and tools. Early efforts may focus on building foundational infrastructure, while more advanced activities involve optimizing performance, scaling solutions, and integrating AI into core business processes.
Data Integrity: Data is the lifeblood of AI. Ensuring data is “AI-ready” requires capabilities beyond traditional data management, including data quality, metadata management, and data observability. Organizations should assess their data readiness for initial use cases, implement plans to address gaps, and invest in long-term data governance to support ongoing AI evolution.
Sequencing and Prioritizing AI Initiatives
A critical aspect of building an AI roadmap is sequencing activities appropriately. Not all organizations need to tackle every activity at once, nor should they. Instead, CIOs should select and sequence actions based on their organization’s specific AI strategy, maturity, and resource constraints. This approach ensures that foundational elements are in place before moving to more advanced initiatives, reducing risk and increasing the likelihood of success.
Goal setting should begin with a clear statement of the choices the organization will make to achieve competitive advantage through AI. This includes defining where AI will be applied—whether to optimize internal operations, enhance customer experiences, or create entirely new business models—and how success will be measured.
Evolving the Organization for AI at Scale
As AI initiatives progress from pilots to scaled deployments, organizations must evolve in tandem. This evolution spans people, processes, and technology. Early-stage efforts may rely on a small group of AI champions, but scaling requires broader engagement, formalized structures, and robust change management.
CIOs should develop a resourcing plan that addresses capability gaps, whether through internal development or external partnerships. Establishing communities of practice and dedicated AI teams can accelerate learning and drive early wins. Over time, these efforts should be institutionalized within a target operating model that supports sustained AI growth.
Data Readiness and Governance
AI-ready data is fundamentally different from data prepared for traditional analytics. Organizations must invest in data preparation, quality assurance, and governance practices that support AI’s unique requirements. This includes not only technical capabilities but also processes for ensuring data privacy, security, and ethical use.
Data observability—monitoring data in production environments—is increasingly important as AI systems move from experimentation to mission-critical applications. Ensuring data integrity and transparency helps maintain trust and supports ongoing optimization.
Measuring and Communicating AI Value
Delivering value from AI is not just about technical achievement; it’s about measurable business outcomes. CIOs must track and communicate the impact of AI initiatives, from productivity gains and cost savings to new revenue streams and enhanced customer experiences.
It’s also important to recognize and articulate the value already being delivered by AI, even as attention shifts to the latest innovations. Demonstrating ongoing value helps sustain executive support and ensures continued investment in AI capabilities.
Building a Culture for Sustainable AI Success
Perhaps the most overlooked aspect of AI adoption is the human factor. As AI becomes more integrated into business processes, employees’ reactions—ranging from enthusiasm to anxiety—can significantly influence outcomes. CIOs should prioritize building a culture that supports AI adoption, encourages learning, and addresses concerns proactively.
Training and upskilling programs, transparent communication, and opportunities for employees to engage with AI initiatives all contribute to a positive culture. A workforce that understands and embraces AI is better positioned to drive innovation and realize the technology’s full potential.
Conclusion
For CIOs and directors, the journey to AI maturity is complex but navigable with a well-structured roadmap. By focusing on the seven key workstreams—strategy, value, organization, people and culture, governance, engineering, and data—organizations can align their AI efforts with business objectives, manage risk, and unlock sustainable value. The roadmap is not static; it must evolve as the organization and the AI landscape change. With a clear vision, disciplined execution, and a commitment to cultural and operational readiness, CIOs can lead their organizations to AI success at scale.